International Chair in Data Science and Explainable Artificial Intelligence
About International Chair in DS & XAI
Towards the goal of developing further studies on Trustworthy, Transparent, Ethical and Human-centered Data Science and Artificial Intelligence, the International Chair in Data Science and Explainable Artificial Intelligence was founded in September 2018, aiming to develop a roadmap that starts from Industry 4.0 to reach Industry 5.0 and beyond which drives sustainability since 2018. Studies about Industry 5.0 will focus on combining human creativity and craftsmanship with the speed, productivity, and consistency of AI systems.
Objectives
- Establishing a framework that exerts magnetism upon the most accomplished global researchers within the domain of Data Science and Artificial Intelligence.
- Coordinating collaborative projects between internationally acclaimed senior researchers and burgeoning junior scientists operating within the precincts of the International Chair.
Vision
International Chair in Data Science and Explainable Artificial Intelligence will establish a Joint Research Insitute of Vietnam & France in close cooperation with other universities in the next 10 years such as University of Kent (UK), University of Liège (Belgium), Polytechnique Montreal (Canada), University of Piraeus (Greece), University of Waterloo (Canada) and etc.
Particularly, the main organizations are the University of Lille, France, and Dong A University (UDA), Vietnam, in which IAD is the key organization and a representative of UDA.
Research topics
- Embedded Artificial Intelligence
- Embedded Federated Learning
- FPGA Design for the Acceleration of AI Algorithms
- Explainability of AI-based prediction
- Trustworthy AI systems
- Explainable Anomaly Detection
- Reinforcement Learning
- Cybersecurity
- Decision Support Systems
- Fairness of AI algorithms
- Privacy-preserving data analytics
- Auditing AI systems
- Distributed/online optimization algorithms
- Coupling optimization/simulation with AI
- Data-driven supply chain network design
- Wearable technologies
- Smart Healthcare
- Smart Manufacturing
- Data Science
- Inverse problems in medical imaging
- Digital Twins in Healthcare
- Digital Twin Application for Production Optimization
- Ethical and Human-centered Artificial Intelligence
Organizational structure:
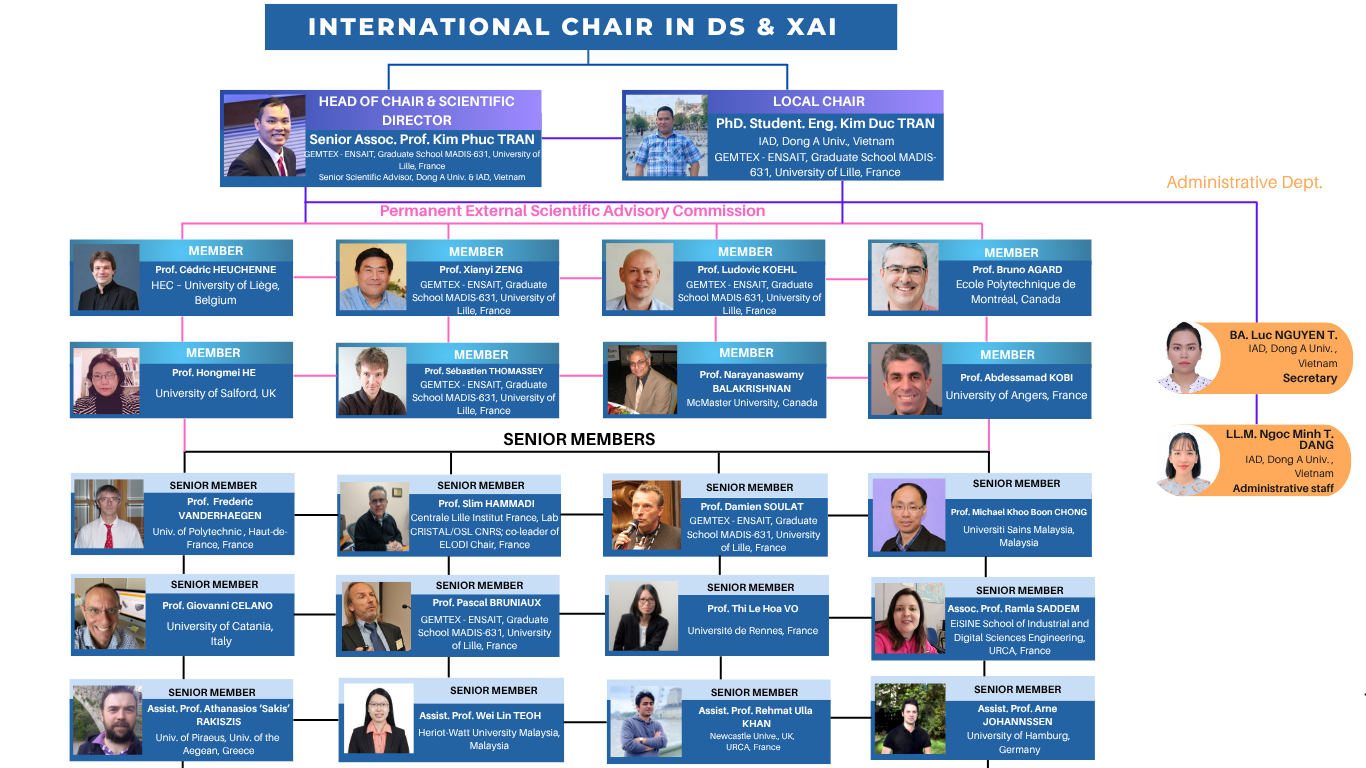
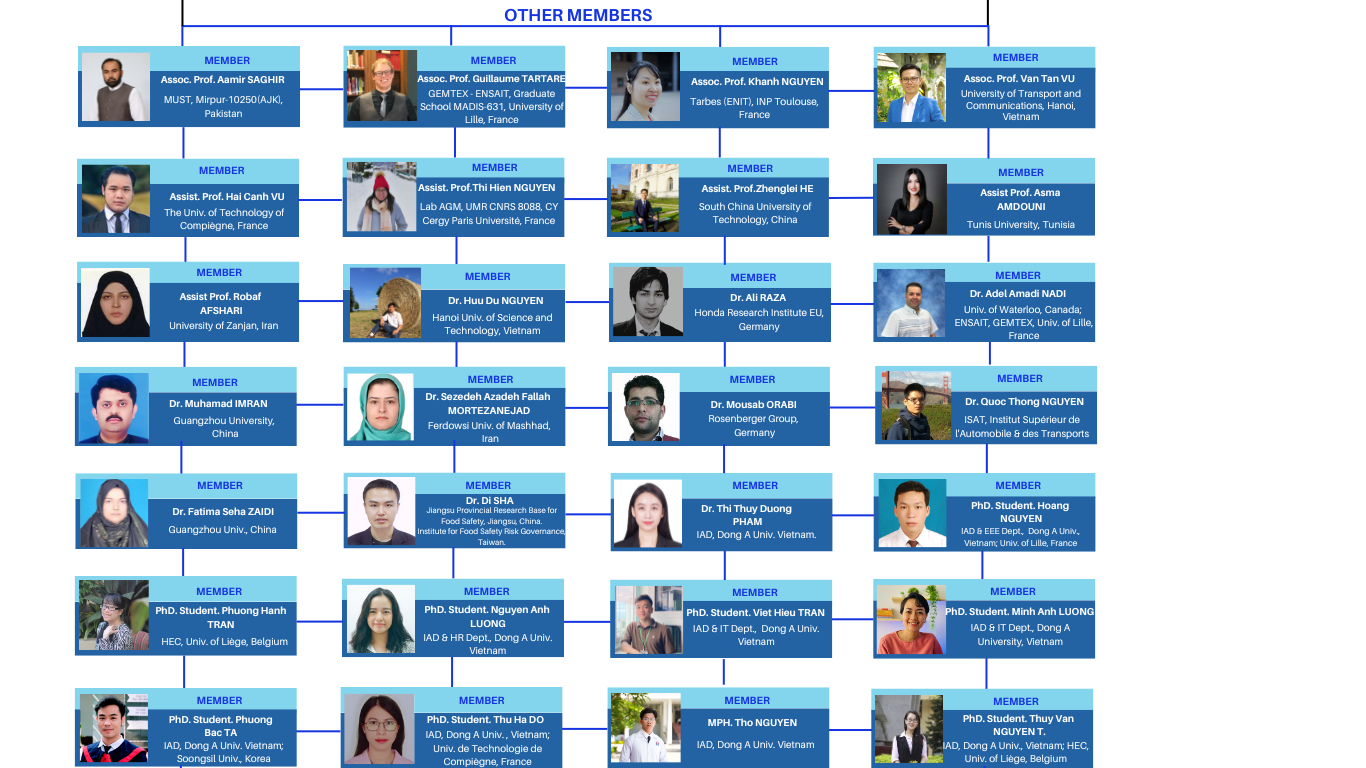

Head of the International Chair
Senior Assoc. Prof. Kim Phuc TRAN
University of Lille, ENSAIT, GEMTEX, France
- Google Scholar: https://scholar.google.fr/citations?hl=en&user=uGv7zzQAAAAJ
- Personal webpage: https://www.gemtex.fr/gemtex-members/phuc-tran/
Biography
Kim Phuc Tran is currently a Senior Associate Professor (Maître de Conférences HDR, equivalent to a UK Reader) of Artificial Intelligence and Data Science at the University of Lille, Graduate School MADIS-631, ENSAIT, & GEMTEX laboratory, France. He received an Engineer's degree and a Master of Engineering degree in Automated Manufacturing. He obtained a Ph.D. in Automation and Applied Informatics at the University of Nantes, and an HDR (Doctor of Science or Dr. Habil.) in Computer Science and Automation at the University of Lille, France. He has published more than 72 papers in peer-reviewed international journals and proceedings of international conferences. He edited 3 books with Springer Nature and Taylor & Francis. He is the Associate Editor, Editorial Board Member, and Guest Editor for several international journals such as IEEE Transactions on Intelligent Transportation Systems and Engineering Applications of Artificial Intelligence. Kim Phuc Tran has supervised 12 Ph.D. students and 3 Postdocs. In addition, as the project coordinator (PI), he conducted a national project about Healthcare Systems with Federated Learning. He has been or is involved (PI, co-PI, or member) in 13 national and European projects. He is an expert and evaluator for the Public Service of Wallonia (SPW-EER), Belgium, the Natural Sciences and Engineering Research Council of Canada, ANRT (Association nationale de la recherche et de la technologie), and CY Cergy Paris University, France. He received the Award for Scientific Excellence (Prime d’Encadrement Doctoral et de Recherche) given by the Ministry of Higher Education, Research and Innovation, France for 4 years from 2021 to 2025 in recognition of his outstanding scientific achievements.
From 2017 until now, he has been the President of the Permanent External Scientific Advisory Board for Dong A University and the International Research Institute for Artificial Intelligence and Data Science (IAD), Danang, Vietnam where he has held the International Chair in Data Science and Explainable Artificial Intelligence. His research interests include Explainable Trustworthy, and Transparent Artificial Intelligence; Ethical, and Human-centered Artificial Intelligence; Safety and Reliability of Artificial Intelligence; Statistical Computing; Intelligent Decision Support Systems; Digital Twins; and Applications of AI, Edge Computing, and Data Science in Industry 5.0.
Local Chair
- PhD. Student. Eng Kim Duc TRAN
Kim Duc Tran is currently Permanent Vice Director of International Research Institute for Artificial Intelligence and Data Science (IAD), Dong A University (UDA), Vietnam. Simultaneously, he is a PhD Student and Data Scientist at Graduate School MADIS-631, GEMTEX & ENSAIT, University of Lille.
Permanent External Scientific Advisory Commission Members:
- Prof. Cédric HEUCHENNE (HEC, University of Liège, Belgium)
- Prof. Xianyi ZENG (ENSAIT, GEMTEX National Laboratory, University of Lille, France)
- Prof. Ludovic KOEHL (GEMTEX National Laboratory, University of Lille, France)
- Prof. Bruno AGARD (Department of Mathematics and Industrial Engineering, Ecole Polytechnique de Montréal, Canada)
- Prof. Hongmei HE (University of Salford, United Kingdom)
- Prof. Sébastien THOMASSEY (ENSAIT, GEMTEX National Laboratory, University of Lille, France)
- Prof. Narayanaswamy BALAKRISHNAN (McMaster University, Canada)
- Prof. Abdessamad KOBI (University of Anger, France)
Senior Members:
- Prof. Frederic VANDERHAEGEN (University of Polytechnic, Haute-de-France, France)
- Prof. Slim HAMMADI (Centrale Lille Institut France, Lab CRISTAL/OSL CNRS, and co-leader of the ELODI Chair)
- Prof. Damien SOULAT (ENSAIT, GEMTEX National Laboratory, University of Lille, France)
- Prof. Michael Khoo Boon CHONG (Universiti Sains Malaysia, Malaysia)
- Prof. Giovanni CELANO (University of Catania, Italy)
- Prof. Pascal BRUNIAUX (ENSAIT, GEMTEX National Laboratory, University of Lille, France)
- Prof. Thi Le Hoa VO (Universite de Rennes, France).
- Assist. Prof. Arne JOHANNSSEN (University of Hamburg, Germany)
- Assoc. Prof. Ramla SADDEM (EiSINE School of Industrial and Digital Sciences Engineering, University of Reims Champagne-Ardenne (URCA) in France)
- Assist. Prof. Rehmat Ullah KHAN (School of Technologies, Cardiff Metropolitan University, UK)
- Assist. Prof. Athanasios ‘Sakis’ RAKITZIS (University of Piraeus, Greece, University of the Aegean)
- Assist. Prof. Wei Lin TEOH (School of Mathematical and Computer Sciences at Heriot-Watt University Malaysia (HWUM), Malaysia)
Members:
- Assoc. Prof. Aamir SAGHIR (Mirpur University of Science and Technology (MUST), Mirpur-10250(AJK), Pakistan)
- Assoc. Prof. Guillaume TARTARE (ENSAIT, GEMTEX National Laboratory, University of Lille, France)
- Assoc. Prof. Khanh NGUYEN (Tarbes (ENIT), INP Toulouse, France)
- Assoc. Prof. Van Tan VU (University of Transport and Communications, Hanoi, Vietnam)
- Assist. Prof. Hai Canh VU (Université de Technologie de Compiègne, Compiègne, France)
- Assist. Prof. Thi Hien NGUYEN (Laboratoire AGM, UMR CNRS 8088, CY Cergy Paris Université, 95000 Cergy, France)
- Assist. Prof. Zhenglei HE (Automation and Intelligent Manufacturing at the State Key Laboratory of Pulp and Paper Engineering, South China University of Technology, China)
- Assist. Prof. Asma AMDOUNI (Tunis University, Tunisia)
- Assist. Prof. Robab AFSHARI (University of Zanjan, Iran)
- Dr. Huu Du NGUYEN (Hanoi University of Science and Technology, Vietnam).
- Dr. Ali RAZA (Honda Research Institute EU, Germany)
- Dr. Adel Ahmadi NADI (University of Waterloo, Canada; ENSAIT, GEMTEX,University of Lille, France)
- Dr. Muhammad IMRAN (Guangzhou University, China)
- Dr. Seyedeh Azadeh Fallah MORTEZANEJAD (Ferdowsi university of Mashhad, Iran)
- Dr. Moussab ORABI (Rosenberger Group, Germany)
- Dr. Quoc Thong NGUYEN (Softt Industries Groupe Highfi, France; IAD, Dong A University, Vietnam)
- Dr. Fatima Sehar ZAIDI (Guangzhou University, China)
- Dr. Di SHA (Jiangsu Provincial Research Base for Food Safety, Jiangsu, China; Institute for Food Safety Risk Governance, Taiwan.)
- Dr. Thi Thuy Duong PHAM (IAD, Dong A University, Vietnam)
- PhD. Student Hoang Nguyen LE (Department of Electrical - Electronics, IAD, Dong A University, Vietnam)
- PhD. Student Phuong Hanh TRAN (HEC, University of Liège, Belgium)
- PhD. Student Phuong Bac TA (Soongsil University, Korea; IAD, Dong A University, Vietnam)
- PhD. Student Thu Ha DO (Université de Technologie de Compiègne, France; IAD, Dong A University)
- MPH. Student Tho NGUYEN (IAD, Dong A University, Vietnam)
- PhD. Student Thi Thuy Van NGUYEN (HEC, University of Liège, Belgium; ENSAIT, GEMTEX, the University of Lille France, IAD, Dong A University, Vietnam)
- PhD. Student. Nguyen Anh LUONG (Dong A University, Vietnam)
- PhD. Student. Viet Hieu TRAN (IT Dept. & IAD, Dong A University, Vietnam)
- PhD. Student Himansui BENIWAL (Indian Institute of Technology Gandhinagar, India)
- PhD. Student. Minh Anh LUONG (IT Dept. & IAD, Dong A University, Vietnam)
- MSc. Dac Hieu NGUYEN (IAD, Dong A University, Vietnam)
- PhD. Student. Long Hai LE (EEE Dept. & IAD, Dong A University, Vietnam)
- Eng. Van Uc NGO (IAD, Dong A University, Vietnam)
- Pharm. Tan Bao Minh NGUYEN (IAD, Dong A University, Vietnam)
International Academic Exchange Program
- 7/2019 - 1/2020: Dr. Huu Du Nguyen conducted a project: Multi-State models: inference and applications as a postdoc researcher under the supervision of Senior Assoc. Prof. Kim Phuc Tran and Prof. Cédric Heuchenne at University of Lille.
- 1/2020 - 3/2021: Dr. Quoc Thong Nguyen carried out a project: Smart manufacturing with Artificial intelligence, IoT, and Big Data as a postdoc researcher under the supervision of Senior Assoc. Prof. Kim Phuc Tran and Prof. Cédric Heuchenne at University of Lille.
- 4/2021-3/2022: Dr. Quoc Thong Nguyen conducted a project: AI and Machine Learning For Fashion Industry under supervisors Prof. Sébastien Thomassey and Senior Assoc. Prof. Kim Phuc Tran.
- 5/2021-5/2022: Dr. Adel NADI conducted a project: Explainable Machine Learning for Anomaly Detection with Applications under supervisor Senior Assoc. Prof. Kim Phuc Tran.
- 10/2022 - 9/2023: Engineer Thu Ha Do conducted the project: Digital Fashion with AI as a postdoc researcher at University of Lille under the supervision of Prof. Xianyi Zeng and Senior Assoc. Prof. Kim Phuc Tran.
- 9/2022 - 9/2025: MS. Thuy Van Nguyen worked at University of Lille as a PhD. Student under the supervision of Assoc. Prof. Kim Phuc Tran and Prof. Cédric Heuchenne on the Project: Anomaly detection in IoT Multivariate Time Series data with Statistical and Machine Learning techniques.
- 01/2024 - 2028: MS. Eng. Hoang Le Nguyen works at University of Lille as a PhD. Student under the supervision of Assoc. Prof. Kim Phuc Tran on the Project: Edge Artificial Intelligence for Smart Factory Applications in Industry 5.0. His PhD funding: Dong A University - International Research Institute for Artificial Intelligence and Data science - scholarship program for excellent lecturers-researchers at XAI &DS chair
Master Student Exchange Program:
- 03/2023 - 05/2023 - (Dong A University, Vietnam and University of Lille, France)
Lukas Viale, Emma Pendolino and Jeanne Bondoni conducted a research project: "How to use ChatGPT in fashion" under the supervision of MBs. Kim Duc Tran, MB. Tho Nguyen, PhD. Student. Thu Ha Do and Dr. Ali Raza.
Milestones
Publications
1. Journals
2025
- J. W. Teoh, W. L. Teoh, M. B. C. Khoo, K. P. Tran, M. H. Lee. (2025). An integrated optimal-GICP design for the SPRT control chart with estimated process parameters based on the average number of observations to signal, Quality Technology & Quantitative Management 22 (1), 84-104
- J. W. Teoh, W. L. Teoh, X. L. Hu, K. P. Tran, D. G. Godase. (2025). A new omnibus SPRT chart for monitoring process mean and variability based on the average number of observations to signal, Journal of Statistical Computation and Simulation 95 (1), 49-69.
- J. Ivars, K. P. Tran, A. R. Labanieh, D. Soulat. (2025). Reliability modeling of tensile properties in recycled carbon fibers: a comparative fit of statistical distributions pre-and post-textile processing, Materials Today Communications, 111818
- T. T. Van Nguyen, C. Heuchenne, K. D. Tran, G. Tartare, K. P. Tran. (2025). SVDD control charts based on MEWMA technique for monitoring compositional data, Computers & Industrial Engineering 201, 110865
- J. A. Bairaktaris, A. Johannssen, K.P. Tran (2025). Security strategies for AI systems in Industry 4.0, Quality and Reliability Engineering International 41 (2), 897-915.
- T. Q. D Pham, K. D. Tran, K. T. P. Nguyen, X. V. Tran, K. P. Tran. (2025). A new framework for prognostics in decentralized industries: Enhancing fairness, security, and transparency through Blockchain and Federated Learning, arXiv preprint arXiv:2503.05725
2024
1. Z. He, Z. Lu, X. Wang, Q. Xiong, K.P. Tran, S. Thomassey, X. Zeng, M. Hong, and Y. Man. (2024). Multiobjective Optimization of Papermaking Wastewater Treatment Processes under Economic, Energy, and Environmental Goals, Environmental Science & Technology, https://doi.org/10.1021/acs.est.4c03460
2. S. Rita, Q.T. Nguyen, A.L. Sandra, K.P. Tran, S. Thomassey. (2024). Evaluating the sales potential of new products using Machine Learning techniques and data collected from mobile applications, International Journal of Clothing Science and Technology, https://doi.org/10.1108/IJCST-07-2023-0099
3. Teoh, J. W., Teoh, W. L., Khoo, M. B., K. P. Tran, & Lee, M. H. (2024). An integrated optimal-GICP design for the SPRT control chart with estimated process parameters based on the average number of observations to signal. Quality Technology & Quantitative Management, 1-21.
4. M. Orabi, K.P. Tran, S. Thomassey, P. Egger. (2024). Anomaly Detection in Smart Manufacturing: An Adaptive Adversarial Transformer based Model, Journal of Manufacturing Systems, https://doi.org/10.1016/j.jmsy.2024.09.021
5. F. S. Zaidi, Hong-Liang Dai, Muhammad Imran, Kim Phuc Tran. (2024). Enhanced Hybrid LSTM and SLAR Modeling for In-Depth Analysis of Temporal and Spatial Patterns in Compositional Data for Environmental Monitoring, Process Safety and Environmental Protection..
6. M. Imran, H.L. Dai, F.S; Zaidi, X. Hu, K.P. Tran, and J. Sun. (2024). Analyzing Out-of-Control Signals of T2 Control Chart for Compositional Data using Artificial Neural Networks, Expert Systems With Applications.
7. S. A. F. Mortezanejad, R. Wang, G. M. Borzadaran, R. Ding, K. P. Tran. (2024). Profile Control Chart based on Maximum Entropy: Accepted-December 2024, REVSTAT-Statistical Journal.
2023
- M. Imran, H.L. Dai, F.S; Zaidi, X. Hu, K.P. Tran, Z. Abbas, & H. Z. Nazir. (2023). Incorporating principal component analysis into Hotelling T2 control chart for compositional data monitoring. Computers & Industrial Engineering, 109755.
- H. D. Nguyen, H. L. Nguyen, N. H. Kieu, V. H. Nguyen, T. H. Truong, & K. P. Tran. (2023). Trans-Lighter: A light-weight federated learning-based architecture for Remaining Useful Lifetime prediction. Computers in Industry, 148, 103888.
- A. Raza, K. P. Tran, L. Koehl, & S. Li. (2023). AnoFed: Adaptive anomaly detection for digital health using transformer-based federated learning and support vector data description. Engineering Applications of Artificial Intelligence, 121, 106051.
- A. A. Nadi, B. S. Gildeh, J. Kazempoor, K. D. Tran, & K. P. Tran (2023). Cost-effective optimization strategies and sampling plan for Weibull quantiles under type-II censoring. Applied Mathematical Modelling, 116, 16-31.
- F.S. Zaidi, H.L Dai, M. Imran, K.P. Tran. (2023). Analyzing Abnormal Pattern of Hotelling T2 Control Chart for Compositional Data using Artificial Neural Networks, Computers & Industrial Engineering, 109254.
- F. S. Zaidi, H.L Dai, M. Imran, K.P. Tran. (2023). Monitoring Autocorrelated Compositional Data Vectors using an Enhanced Residuals Hotelling T2 Control Chart, Computers & Industrial Engineering.
- A. Saghir, X. Hu, K.P. Tran, Z. Song. (2023). Optimal design and evaluation of adaptive EWMA monitoring schemes for Inverse Maxwell distribution, Computers & Industrial Engineering.
2022
- H. D. Nguyen, K. P. Tran, S.Thomassey & M. Hamad. (2021). Forecasting and Anomaly Detection approaches using LSTM and LSTM Autoencoder techniques with the applications in supply chain management. International Journal of Information Management, 57, 102282.
- H. D. Nguyen, A. A. Nadi, K. D. Tran, P. Castagliola, G. Celano, & K. P. Tran. (2022). The Shewhart-type RZ control chart for monitoring the ratio of autocorrelated variables. International Journal of Production Research, 1-26.
- H. T. Truong, B. P. Ta, Q. A. Le, D. M. Nguyen, C. T. Le, H. X. Nguyen, H.T. Do, H.T. Nguyen & K. P. Tran. (2022). Light-weight federated learning-based anomaly detection for time-series data in industrial control systems. Computers in Industry, 140, 103692.
- A. Raza, K. P. Tran, L. Koehl & S. Li. (2022). Designing ECG monitoring healthcare system with federated transfer learning and explainable AI. Knowledge-Based Systems, 236, 107763.
- Z. He, K. P. Tran, S. Thomassey, X. Zeng, J. Xu & C. Yi. (2022). Multi-objective optimization of the textile manufacturing process using deep-Q-network based multi-agent reinforcement learning. Journal of Manufacturing Systems, 62, 939-949.
- A. Raza, S. Li , K. P. Tran, L. Koehl, S. Li & K. Ludovic. (2022). Detection of Poisoning Attacks with Anomaly Detection in Federated Learning for Healthcare Applications: A Machine Learning Approach. arXiv preprint arXiv:2207.08486
- R. Afshari, A. A. Nadi, A. Johannssen, N. Chukhrova & K. P. Tran. (2022). The effects of measurement errors on estimating and assessing the multivariate process capability with imprecise characteristic. Computers & Industrial Engineering, 108563.
2021
- T. H. Truong, P. B. Ta, M. L. Dao, D. L. Tran, M. D. Nguyen, A.Q. Le, T. C. Le, D. T. Bui & K. P. Tran. (2021). Detecting cyberattacks using anomaly detection in industrial control systems: A Federated Learning approach. Computers in Industry, 132, 103509.
- K. D. Tran, A. A. Nadi, T. H. Nguyen & K. P. Tran. (2021). One-sided Shewhart control charts for monitoring the ratio of two normal variables in short production runs. Journal of Manufacturing Processes, 69, 273-289.
- H. D. Nguyen, K. P. Tran & K. D. Tran. (2021). The effect of measurement errors on the performance of the Exponentially Weighted Moving Average control charts for the Ratio of Two Normally Distributed Variables. European Journal of Operational Research, 293(1), 203-218.
- Z. He, K. P. Tran, S. Thomassey, X.Zeng, J.Xu & C. Yi. (2021). A deep reinforcement learning based multi-criteria decision support system for optimizing textile chemical process. Computers in Industry, 125, 103373.
- T. H. Truong, P. B. Ta, M. L. Dao, T. D. Bui, D. L. Tran & K. P. Tran. (2021). Lockedge: Low-complexity cyberattack detection in iot edge computing. IEEE Access, 9, 29696-29710.
- Q. T. Nguyen, V. Giner-Bosch, K. D. Tran, C. Heuchenne & K. P. Tran. (2021). One-sided variable sampling interval EWMA control charts for monitoring the multivariate coefficient of variation in the presence of measurement errors. The International Journal of Advanced Manufacturing Technology, 115(5), 1821-1851.
- A. Raza, K. P. Tran, L. Koehl, S. Li, X. Zeng, & K. Benzaidi. (2021). Lightweight Transformer in Federated Setting for Human Activity Recognition. arXiv preprint arXiv:2110.00244.
2020
- H. D. Nguyen, A. A. Nadi, K. D. Tran, P. Castagliola, G. Celano, & K. P. Tran. (2022). The Shewhart-type RZ control chart for monitoring the ratio of autocorrelated variables. International Journal of Production Research, 1-26.
- H. D. Nguyen, K. P. Tran, G. Celano, P. E. Maravelakis, and P. Castagliola. (2020). On the effect of the measurement error on Shewhart t and EWMA t control charts. The International Journal of Advanced Manufacturing Technology, Vol 107, no. 9 (2020): 4317-4332
- H. D. Nguyen, and K. P. Tran, Effect of the measurement errors on two one‐sided Shewhart control charts for monitoring the ratio of two normal variables. Quality and Reliability Engineering International, Vol 36, no. 5 (2020): 1731-1750
- P. H. Tran, C. Heuchenne, H. D. Nguyen, and H. Marie, "Monitoring coefficient of variation using one-sided run rules control charts in the presence of measurement errors.", Journal of Applied Statistics, Vol 48, issue 12 (2020): 2178-2204
2019
- K.P. Tran, H.D. Nguyen, P.H. Tran, C. Heuchenne, ”On the Performance of CUSUM control charts for monitoring the Coefficient of Variation with Measurement Errors”, The International Journal of Advanced Manufacturing Technology, Vol 104 (2019): 5-8, 1903-1917
- Q. T. Nguyen, K. P. Tran, P. Castagliola, G. Celano, and S. Lardjane, “One-sided synthetic control charts for monitoring the multivariate coefficient of variation.”, Journal of Statistical Computation and Simulation, Vol 89, no. 10 (2019): 1841-1862
- H. D. Nguyen, K. P. Tran, and H. L. Heuchenne, "CUSUM control charts with variable sampling interval for monitoring the ratio of two normal variables.", Quality and Reliability Engineering International, Vol 36, no. 2 (2019): 474-497
- H. D. Nguyen, K. P. Tran, and T. N. Goh, "Variable sampling interval control charts for monitoring the ratio of two normal variables.", Journal of Testing and Evaluation, Vol 48, no. 3 (2019): 2505-2529
- H. D. Nguyen, and E. Gouno, "Maximum likelihood and Bayesian inference for common-cause of failure model.", Reliability Engineering and System Safety, Vol 182 (2019): 56-62, ISSN 0951-8320
- H. D. Nguyen, Q. T. Nguyen, K. P. Tran, and D. P. Ho, "On the performance of VSI Shewhart control chart for monitoring the coefficient of variation in the presence of measurement errors.", The International Journal of Advanced Manufacturing Technology, Vol 104, no. 1 (2019): 211-243
- K. P. Tran, H. D. Nguyen, P. H. Tran, and C. Heuchenne, "On the performance of CUSUM control charts for monitoring the coefficient of variation with measurement errors.", The International Journal of Advanced Manufacturing Technology, Vol 104, no. 5 (2019): 1903-1917
- P.H. Tran, K.P. Tran, and A.C. Rakitzis, “A Synthetic median control chart for monitoring the process mean with measurement errors”, Quality and Reliability Engineering International, Vol 35, issue 4 (2019): 1100-1116
- Q. T. Nguyen, K. P. Tran, H. L. Heuchenne, T. H. Nguyen, and H. D. Nguyen, "Variable sampling interval Shewhart control charts for monitoring the multivariate coefficient of variation.", Applied Stochastic Models in Business and Industry, Vol 35, issue 5 (2019): 1253-1268
- H. D. Nguyen, K. P. Tran, and C. Heuchenne, "Monitoring the ratio of two normal variables using variable sampling interval exponentially weighted moving average control charts.", Quality and Reliability Engineering International, Vol 35, issue 1 (2019): 439-460
- K. P. Tran, P. Castagliola, T. H. Nguyen, and A. Cuzol, "Design of a variable sampling interval EWMA median control chart", International Journal of Reliability, Quality and Safety Engineering, Vol. 26, no. 5 (2019): 1950021
2. Chapters
2025
- A. Saghir, K. D. Tran, K. P. Tran. (2025). Explainable Machine Learning based Control Charts for High-Dimensional Non-Stationary Time Series Data in IoT Systems: Challenges, Methods, and Future Directions, Computational Techniques for Smart Manufacturing in Industry 5.0: Methods and Application, 166 184. DOI:10.1201/9781003399322-7
2024
- H. C. Vu, K. D. Tran, V. H. Tran, K. P. Tran. (2024), Predictive Maintenance Optimization Based on Genetic Algorithms for Future Industrial Systems, Artificial Intelligence for Safety and Reliability Engineering: Methods, Applications, and Challenges, (25-47), Springer Nature Switzerland.
- M. Orabi, K. P. Tran, S. Thomassey, P. Egger. (2024), Anomaly Detection for Catalyzing Operational Excellence in Complex Manufacturing Processes: A Survey and Perspective, Artificial Intelligence for Safety and Reliability Engineering: Methods, Applications, and Challenges, (49-78), Springer Nature Switzerland.
- D. H Nguyen, T. H. Nguyen, K. D. Tran, K. P. Tran. (2024), Physics-Informed Machine Learning for Industrial Reliability and Safety Engineering: A Review and Perspective, Artificial Intelligence for Safety and Reliability Engineering: Methods, Applications, and Challenges, (5-23), Springer Nature Switzerland.
- LH Nguyen, KD Tran, X Zeng, KP Tran. (2024). Human-Centered Edge Artificial Intelligence for Smart Factory Applications in Industry 5.0: A Review and Perspective, Artificial Intelligence for Safety and Reliability Engineering: Methods, Applications, and Challenges, (79-100), Springer Nature Switzerland.
- T. H. Nguyen, A. Saghir, K. D. Tran, D. H. Nguyen, N. A. Luong, K. P. Tran. (2024). Safety and Reliability of Artificial Intelligence Systems, Artificial Intelligence for Safety and Reliability Engineering: Methods, Applications, and Challenges, (185-199), Springer Nature Switzerland.
- K. P. Tran. (2024). Introduction to Artificial Intelligence for Safety and Reliability Engineering, Artificial Intelligence for Safety and Reliability Engineering: Methods, Applications, and Challenges, (185-199), Springer Nature Switzerland.
- T. Nguyen, D. H. Nguyen, Q. T. Nguyen, K. D. Tran, K. P. Tran. (2024) Human-Centered Edge AI and Wearable Technology for Workplace Health and Safety in Industry 5.0, Artificial Intelligence for Safety and Reliability Engineering: Methods, Applications, and Challenges, (171-183), Springer Nature Switzerland.
2023
- H. D. Nguyen, and K. P. Tran. (2023), "Artificial Intelligence for Smart Manufacturing in Industry 5.0: Methods, Applications, and Challenges". Artificial Intelligence for Smart Manufacturing: Methods, Applications, and Challenges, 5-33. Springer, https://doi.org/10.1007/978-3-031-30510-8_2
- H. D. Nguyen, P. H. Tran, T. H. Do, and K. P. Tran. (2023), Quality Control for Smart Manufacturing in Industry 5.0. In Artificial Intelligence for Smart Manufacturing: Methods, Applications, and Challenges, 35-64. Cham: Springer International Publishing, https://doi.org/10.1007/978-3-031-30510-8_3
- P. H. Tran, H. D. Nguyen, C. Heuchenne, and K. P. Tran. (2023), Monitoring Coefficient of Variation Using CUSUM Control Charts. In Springer Handbook of Engineering Statistics (pp. 333-360). London: Springer London, https://doi.org/10.1007/978-1-4471-7503-2_18
- T. Nguyen, K. D. Tran, A. Raza, Q. T. Nguyen, H. M. Bui, and K. P. Tran. (2023), Wearable Technology for Smart Manufacturing in Industry 5.0. In Artificial Intelligence for Smart Manufacturing: Methods, Applications, and Challenges (pp. 225-254). Cham: Springer International Publishing, https://doi.org/10.1007/978-3-031-30510-8_11
- T. P. Bac, D. T. Ha, K. D. Tran, and K. P. Tran. (2023), Explainable Articial Intelligence for Cybersecurity in Smart Manufacturing. In Artificial Intelligence for Smart Manufacturing: Methods, Applications, and Challenges (pp. 199-223). Cham: Springer International Publishing, https://doi.org/10.1007/978-3-031-30510-8_10
- D. T. Ha, T. P. Bac, K. D. Tran, and K. P. Tran. (2023), Efficient and Trustworthy Federated Learning-Based Explainable Anomaly Detection: Challenges, Methods, and Future Directions. In Artificial Intelligence for Smart Manufacturing: Methods, Applications, and Challenges (pp. 145-166). Cham: Springer International Publishing, https://doi.org/10.1007/978-3-031-30510-8_8
2022
- P. H. Tran, A. Ahmadi Nadi, T. H. Nguyen, K. D. Tran & K. P. Tran. (2022). Application of Machine Learning in Statistical Process Control Charts: A Survey and Perspective. In Control Charts and Machine Learning for Anomaly Detection in Manufacturing (pp. 7-42). Springer, Cham.
- H. D. Nguyen, K. P. Tran, P. Castagliola & F. M. Megahed. (2022). Enabling Smart Manufacturing with Artificial Intelligence and Big Data: A Survey and Perspective. In Advanced Manufacturing Methods (pp. 1-26). CRC Press.
3. Conferences
- TTV Nguyen, KP Tran, C Heuchenne, G Tartare. (2024). Poster" Anomaly Detection for IoT multivariate time series data with statistical and machine learning techniques, JRDMA 2024". 10th edition of the Journée Régionale des Doctorants en sciences de la Mer et de l’Automatique (JRDMA).
- A. Saghir, H. Beniwal, K. D. Tran, A. Raza, L. Koehl, X. Zeng & K. P. Tran. (2024). Explainable Transformer-Based Anomaly Detection for Internet of Things Security Check for updates. The Seventh International Conference on Safety and Security with IoT: SaSeIoT 2023.
- T. H. Do, X. H. Nguyen, V. H. Nguyen, H. D. Nguyen, T. H. Truong & K. P. Tran. (2022, June). Explainable Anomaly Detection for Industrial Control System Cybersecurity. Proceedings of The IFAC 10th conference on MANUFACTURING MODELING, MANAGEMENT AND CONTROL, Nantes, France.
- F. Ouedraogo, C. Heuchenne, Q. T. Nguyen & H. Tran. (5–9 Sept, 2021). Data-Driven Approach for Credit Card Fraud Detection with Autoencoder and One-Class Classification Techniques, Proceedings of the Advances in Production Management Systems. Artificial Intelligence for Sustainable and Resilient Production Systems (APMS 2021), Nantes, France, Part I, https://doi.org/10.1007/978-3-030-85874-2_4
- K.P. Tran H. D. Nguyen, Q. T. Nguyen, and W. Chattinnawat. (2018) One-Sided synthetic control charts for monitoring the Coefficient of Variation with Measurement Errors, Proceedings of The IEEE International Conference on Industrial Engineering and Engineering Management, Bangkok, Thailand.
- K.P. Tran, P. Castagliola, T.H. Nguyen and A. Cuzol. (2018). The Efficiency of the VSI Exponentially Weighted Moving Average Median Control Chart. 24nd ISSAT International Conference on Reliability and Quality in Design. Toronto, Ontario, Canda.
4. Books
Embedded Artificial Intelligence Platforms
Federated learning-based anomaly detection testbed
Light-weight federated learning-based anomaly detection for time-series data in industrial control systems
Federated learning-based anomaly detection is developed and studied by the collaboration of Future Internet Laboratory – HUST, IAD – UDA, and University of Lille – France.
Main goal is to design an AD system (FATRAF) that has a fast training time and is light weight to accommodate frequent learning update, while still either retaining the same or improving the detection performance in comparison with some existing AD solutions for ICSs in the literature.
Federated learning (FL)-based anomaly detection architecture was proposed a new hybrid solution of Autoencoder, Transformer and Fourier transform operating in a chain to enhance the detection performance, whilst reducing the training time and being more lightweight for more feasible deployment over edge devices of an IIoT-based industrial control system.
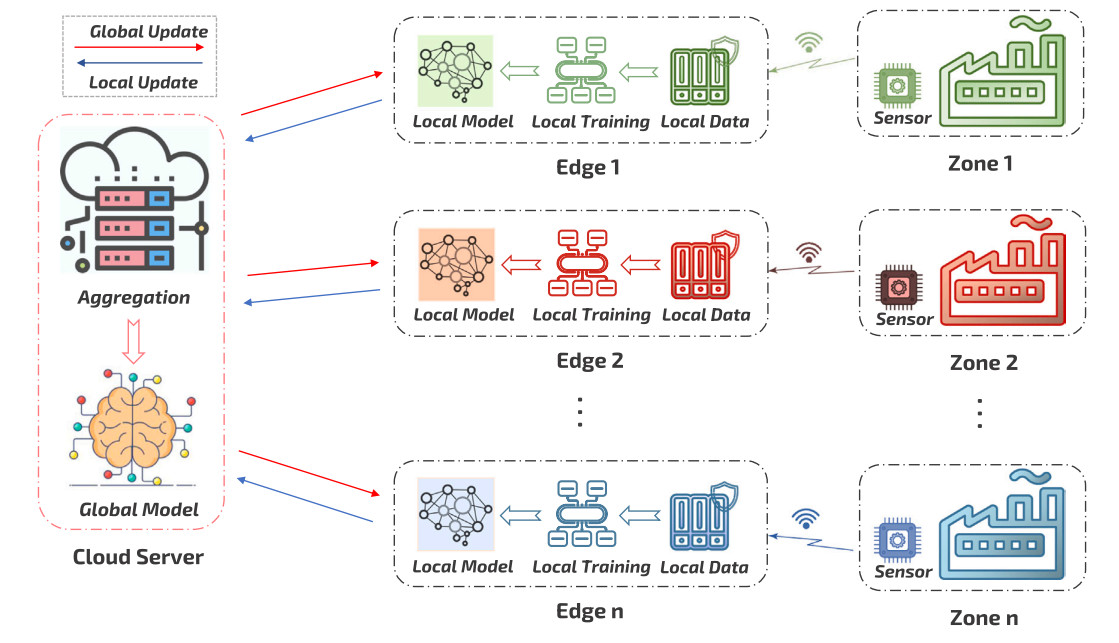
Figure 1. Federated learning (FL) – based anomaly detection architecture (FATRAF)
FATRAF comprises two main components:
- Edge sites: In an IIoT-based smart factory monitored by industrial control systems, there are various manufacturing zones, in which sensor systems are installed to gather readings that signify operating states over time. Subsequently, the time-series data, as the local data, is transmitted wirelessly to edge devices in the vicinity of the corresponding manufacturing zones. Designated to monitor anomalies, these edge devices employ the local data as inputs for training its own local anomaly detection model - ATRAF (AE-Transformer-Fourier learning model). This deployment allows detecting anomalies timely right at the edge sites, making use of the computing capacity of edge devices, and distributing heavy computation tasks that could overload the cloud server.
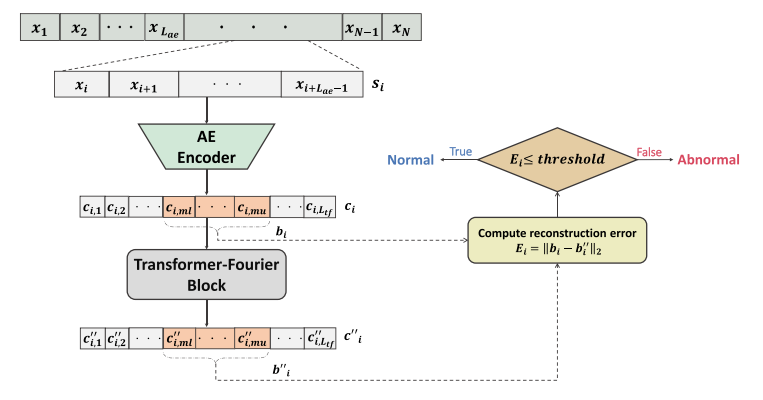
Figure 2. ATRAF learning model operation during the testing process
- Cloud Server: The cloud server undertakes two primary functions: system initialization and aggregation of local models sent from different edges. The whole process of model aggregation and global model updating down to all local models are called Federated Learning.
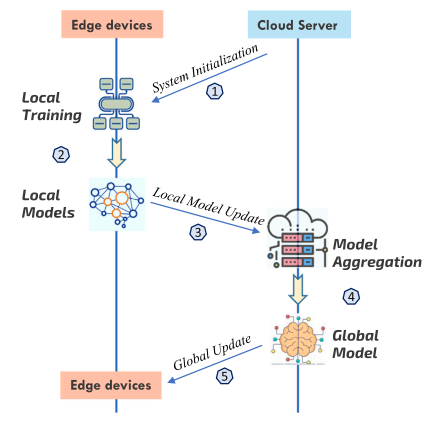
The procedure of FATRAF can be summarized into the following key stages:
- System Initialization: At the beginning, the cloud server establishes a global model with specific learning parameters and sending it to each edge device of corresponding edge site.
- Local Training: After receiving the initial configuration, by utilizing the on-site data collected from sensors, edge devices conduct a local training process for their Autoencoder blocks. Subsequently, at each edge site, the local training data is fed into the encoder part of the trained Autoencoder model so as to obtain corresponding embeddings or code sequences. These code sequences are then applied to train the Transformer-Fourier block.
- Local Model Update: After the local training, each edge device sends the learnable parameters of the Transformer-Fourier block wtr+1 to the cloud server for aggregation. Specifically, wtr+1 includes weight and bias matrices from WQ, WK, WV, WZ nodes in the Masked Self-attention sublayer and all linear transformations in the Position-wise Feedforward sublayer along with Final Feedforward Network.
- Model Aggregation: After deriving trained weights wtr+1 from all edge clients, the cloud server federates them and constructs a new global model version.
- Global Update: Finally, the cloud server broadcasts back the new configuration wt+1 to each edge device as to update the local models of Transformer-Fourier block. In the subsequent learning rounds, edge devices use the updated models to continue training and the communication process will repeat in order to optimize the local Transformer-Fourier models until the global learning model converges.
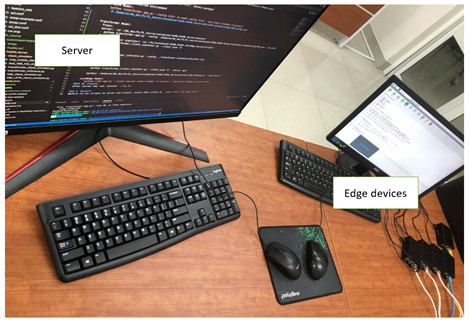 |
Essential devices for testbed in Federated-learning mode, the experiment is conducted using:
- CPU Intel Core i9-12900K
- GPU ROG STRIX RTX3090
- Edge device: raspberry pi 4 (quantify: 05)
|
Designing ECG Monitoring Healthcare System with Federated Transfer Learning and Explainable AI
Federated Transfer Learning and Explainable AI is developed and studied by the collaboration of ENSAIT, GEMTEX Laboratory – University of Lille, IAD – UDA, and School of Computing & Institute of Cyber Security for Society (iCSS), University of Kent, UK.
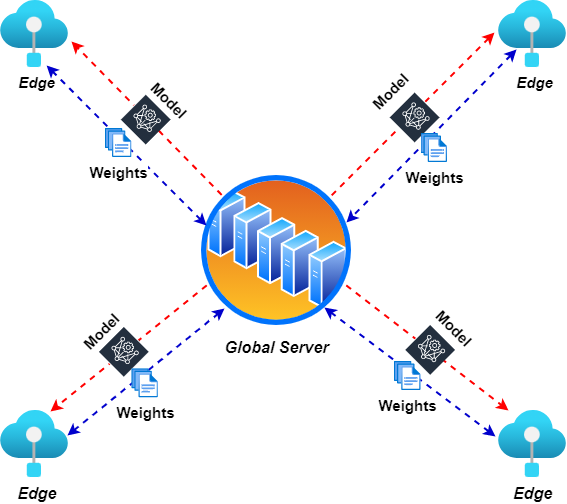
Figure 1. Architecture of Federated Learning
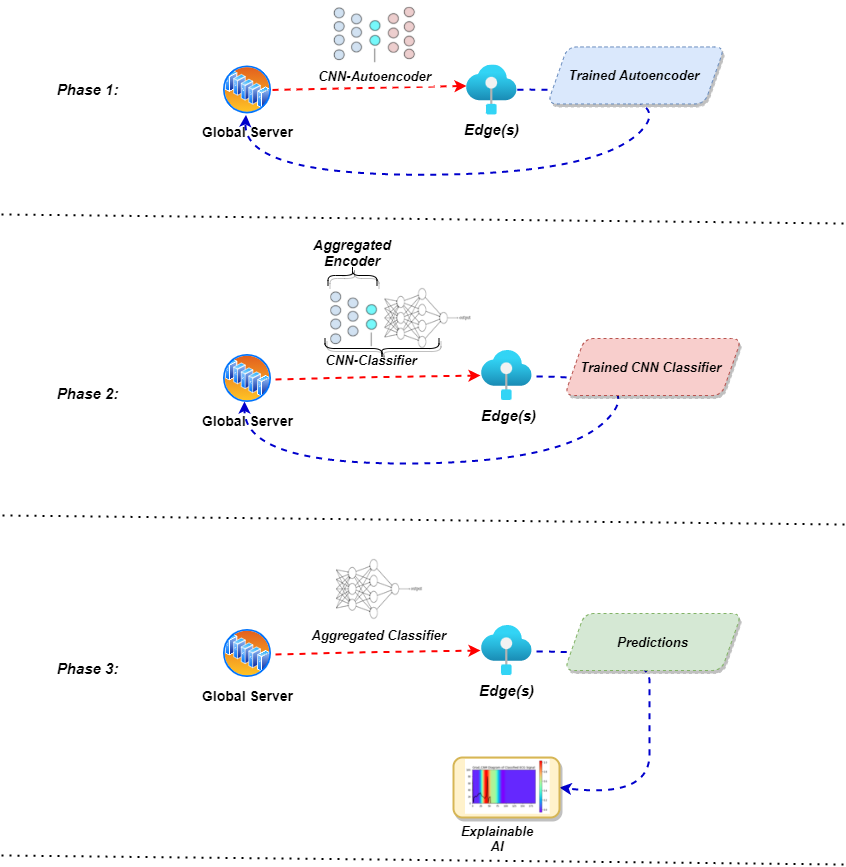
Figure 2. An overview of the proposed framework
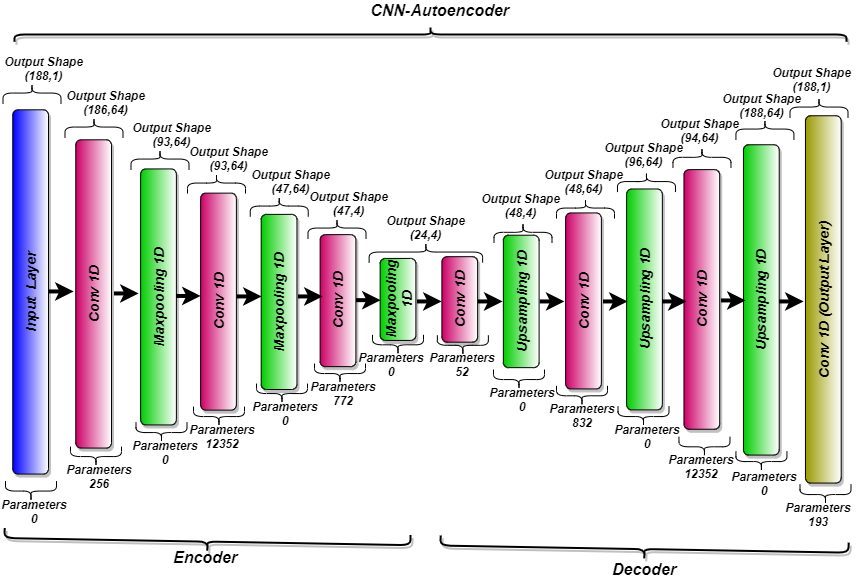
Figure 3. The architecture of the proposed denoising autoencoder
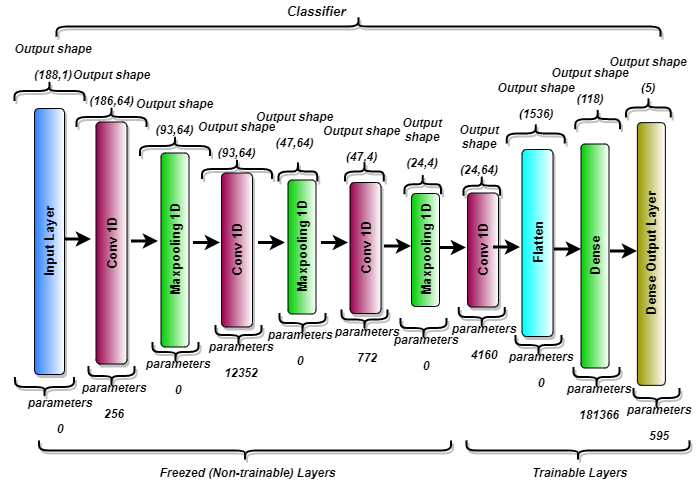
Figure 4. The architecture of the proposed denoising autoencoder
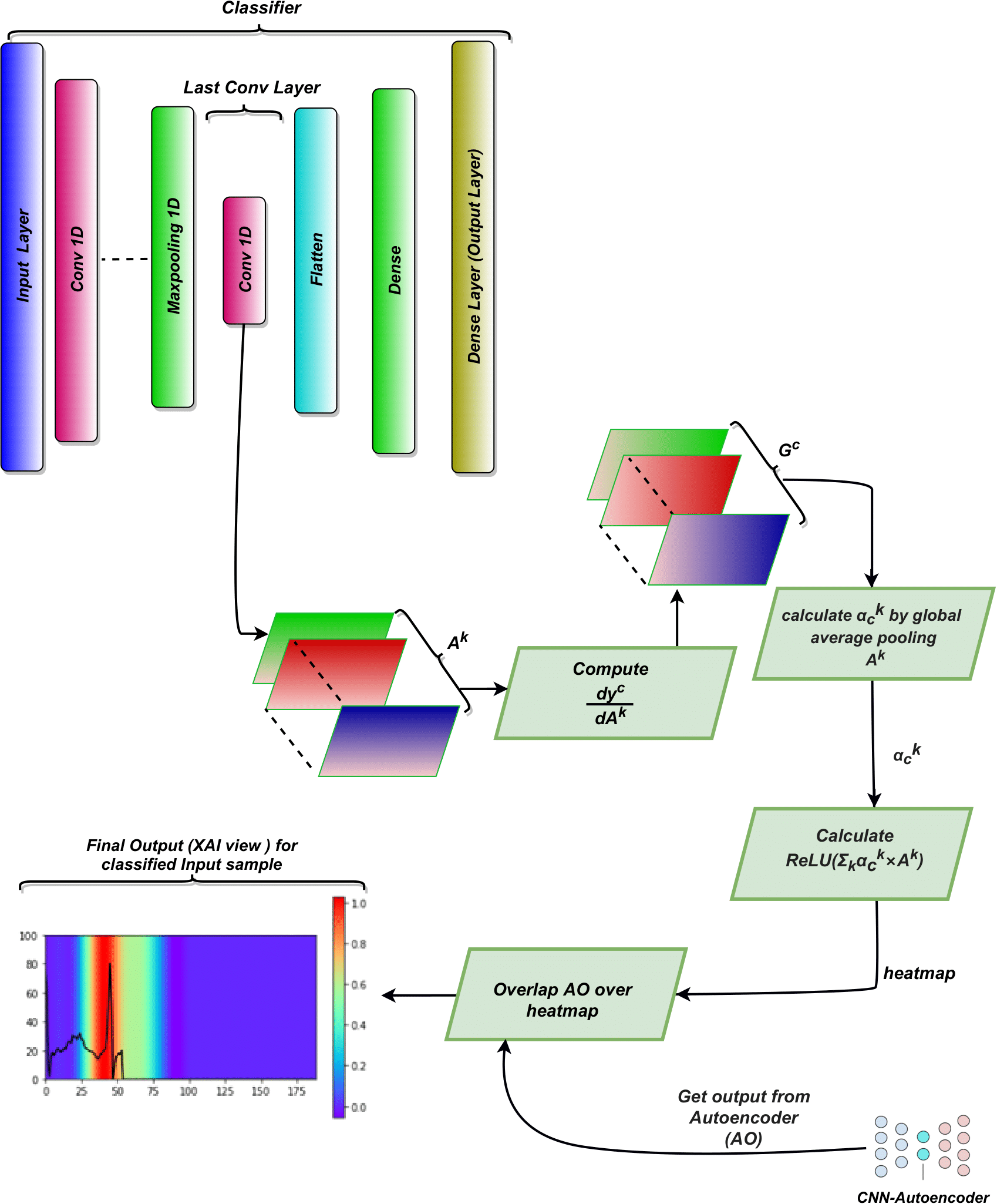
Figure 5. Overview of the proposed XAI module in our framework
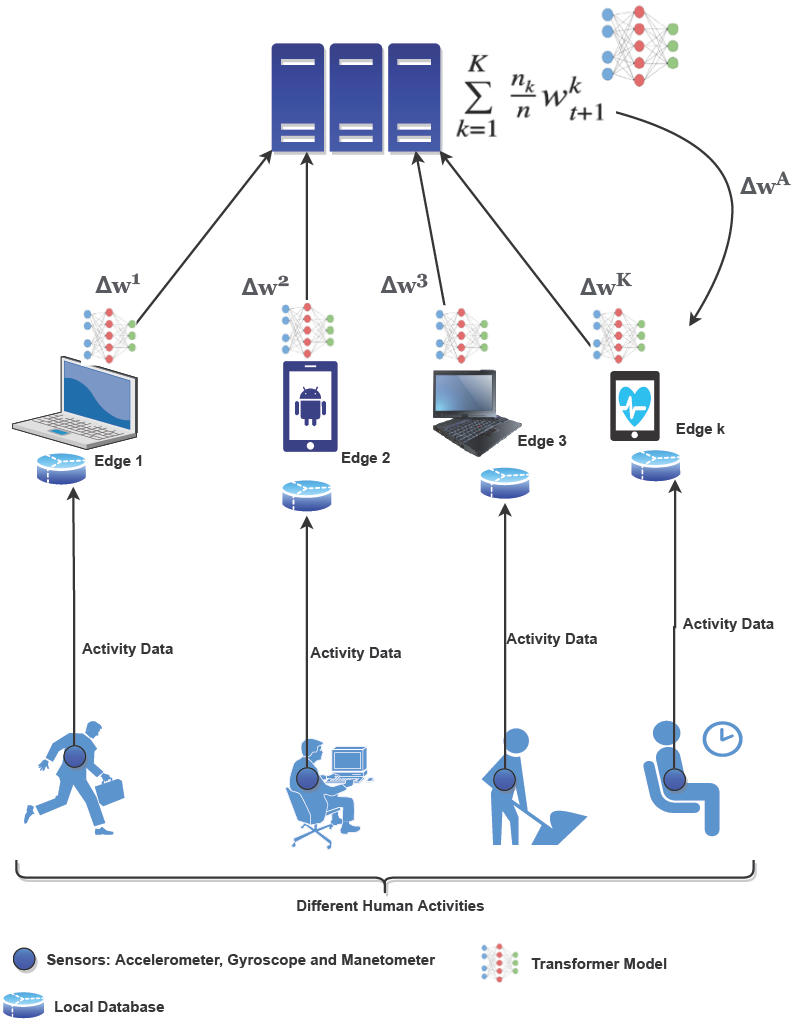
Figure 6. Federated learning architecture in Healthcare system (i.e ECG)
Besides, we apply FL architecture in Heathcare (i.e. ECG) system. First phase, we develop methodology based on sample data. Then, we tend to collect data and extend our system by using IoT sensor (i.e. ECG) in person.
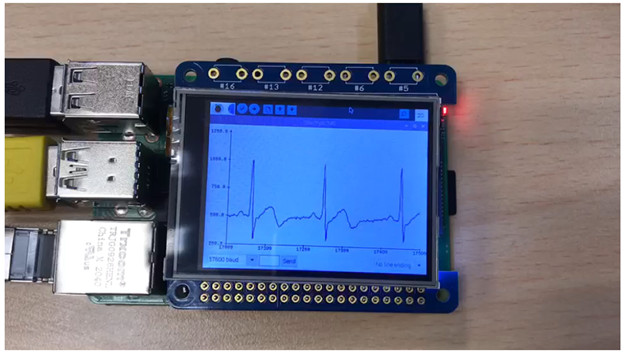
Figure 7. ECG signal displayed in IoT device
FPGA Platform Based on Xilinx Zynq UltraScale and MPSoC XCZU15EG FPGA Development Board
We intend this as a first step toward building a future FPGA-focused HPC community and ecosystem in Vietnam and the EU. We will run training events and develop materials to ensure accessibility. The physics-based testbed at EPCC's Advanced Compute Facility is publicly available.
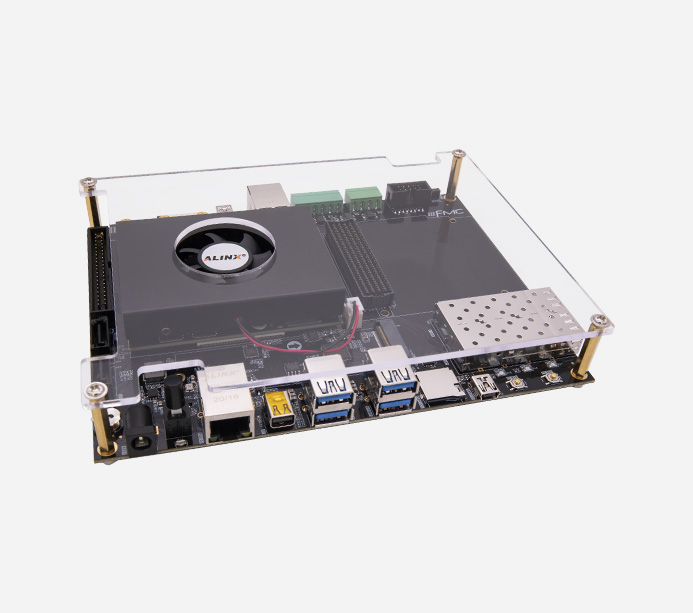
Figure 8. ALINX AXU15EG FPGA
Federated learning model, collects data on engine temperature, vibration and sound to anomaly detection occurring to the engine
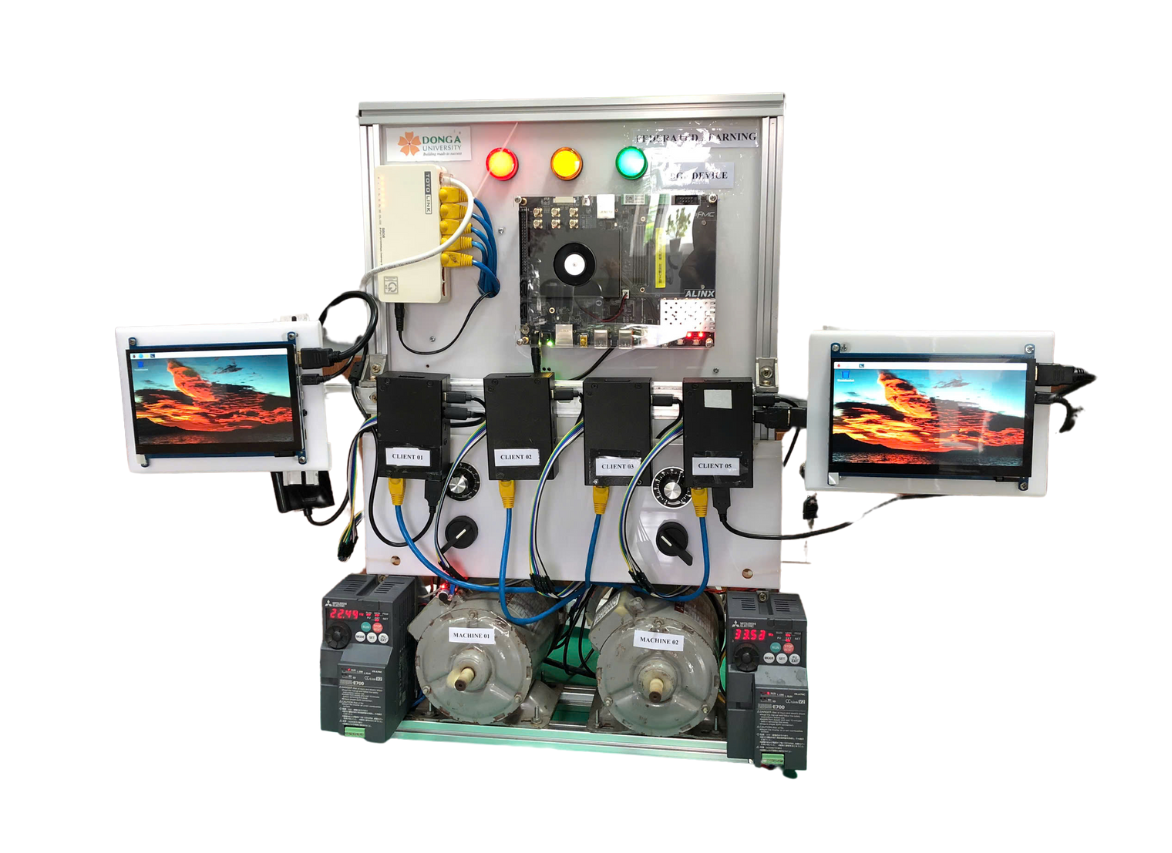
Contact
Location: Room 311B, Dong A University, 33 Xo Viet Nghe Tinh street, Hai Chau district, Da Nang city, Viet Nam
Email:
Head of International Chair
Senior Associate Professor Kim Phuc Tran: kimphuc.tran@univ-lille.fr
Members
MBA. Kim Duc Tran - Local Chair: duckt@donga.edu.vn
MD. Tho Nguyen - Head of the international Unit of IAD, Coordinator & Researcher: thon@donga.edu.vn